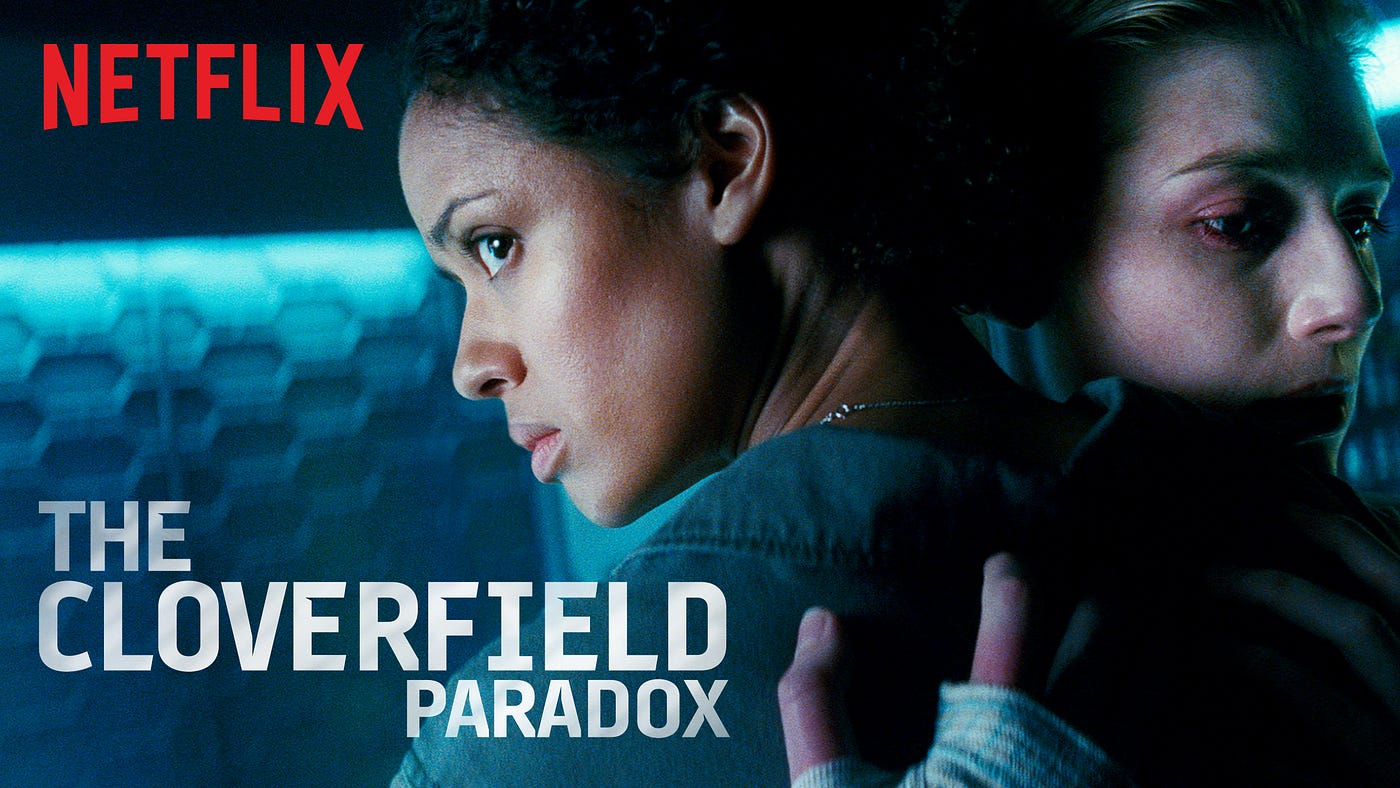
At Netflix, the Content Platform Engineering and Global Product Creative teams know that imagery plays an incredibly important role in how viewers find new shows and movies to watch. We take pride in surfacing the unique elements of a story that connect our audiences to diverse characters and story lines. As our Original content slate continues to expand, our technical experts are tasked with finding new ways to scale our resources and alleviate our creatives from the tedious and ever-increasing demands of digital merchandising. One of the ways in which we do this is by harvesting static image frames directly from our source videos to provide a more flexible source of raw artwork.
The Business Case
Merchandising stills are static video frames taken directly from the source video content used to broaden the reach of a title on the Netflix service. Within a single one-hour episode of Stranger Things, there are nearly 86,000 static video frames.
Traditionally, these merchandising stills are selected by human curators or editors, and require an in-depth expertise of the source content that they’re intended to represent. We know through A/B testing that we can effectively drive increased viewing from expected and unexpected audience groups by exploring as many representations of a title as possible. When it comes to title key art, we like to test many artistic representations of a title in order to find the “right” artwork for the right audience. While this presents an exciting opportunity for innovation and testing, it simultaneously presents a very challenging expectation to scale this experience across every title in our growing global catalog.
تعليقات: 0
إرسال تعليق